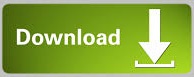

Last, and perhaps most exciting, because deep learning models are not given explicit instructions on features of interest in an image, well-trained models may be able to extract patterns that are beyond human perception ( 12). Whereas rules-based software applications are rigid, deep learning can achieve higher prediction and classification accuracy with increasing amounts of data ( 11). To put it more concretely, rather than instructing software to identify hemorrhage by looking for increased attenuation on head CT images, deep learning allows us to provide the algorithm with examples of head CT scans with and without hemorrhage and allows the algorithm to learn the relevant imaging features to identify the differences. The key difference between deep learning and other programming techniques is that in deep learning, an algorithm can automatically extract features and learn patterns in datasets without being given an explicit set of rules or list of features ( 10). Deep learning is the most common type of AI adopted for image interpretation tasks, including those in radiology ( 9). AI encompasses a group of techniques that simulate human intelligence in machines ( 9). The term AI has been used extensively, and occasionally loosely, in the past several years as a method to drive marketing and sales of medical software.

The authors do not name or endorse any specific companies, and therefore we do not report on any specific performance claims by vendors.
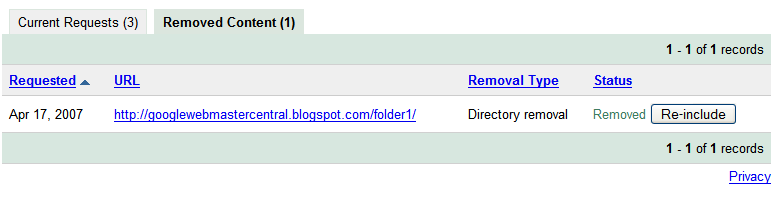
Although there are many companies that focus on nonimaging-related tasks such as automatic structured reporting or billing, in this report we will concentrate on tasks that are primarily focused on image interpretation. By the end of this report, we hope the reader will gain a deeper understanding of radiology AI technology, its potential value-adds to their practice, and identify key points and pitfalls that should be considered before purchasing. The goal of this report is to briefly define the fundamental concepts and terminology in AI, highlight key stakeholders and important considerations in purchasing or adopting radiology AI software, and outline some of the current software offerings by AI companies. In contrast, the majority of the health care record is unstructured, and key information and diagnoses may exist in different areas. Radiology is an appealing area of focus for AI because radiology images consist of a specified set of standard examination types and views, as well as some degree of structured reporting, depending on the institution’s guidelines. The 2019 Radiological Society of North America (RSNA) Annual Meeting had 173 self-identified radiology AI companies. With this progress in technology, there has been a concurrent explosion of medical AI companies aiming to solve problems in radiology. AI has also been leveraged extensively for imaging tasks such as identification of intracranial hemorrhage at head CT ( 6) and identifying diabetic retinopathy at fundoscopic examinations ( 7, 8). In health care, AI has been used for a variety of tasks, including predicting hospital readmission rates ( 1– 3), intensive care unit mortality ( 4), and identifying patients in intensive care units at risk for sepsis ( 5). Artificial intelligence (AI) has demonstrated tremendous advancements in recent years for learning patterns from large-scale data, tackling problems like facial recognition and self-driving vehicles.
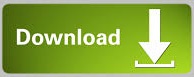